Machine Learning Services
Machine Learning for Better Public Service Delivery.
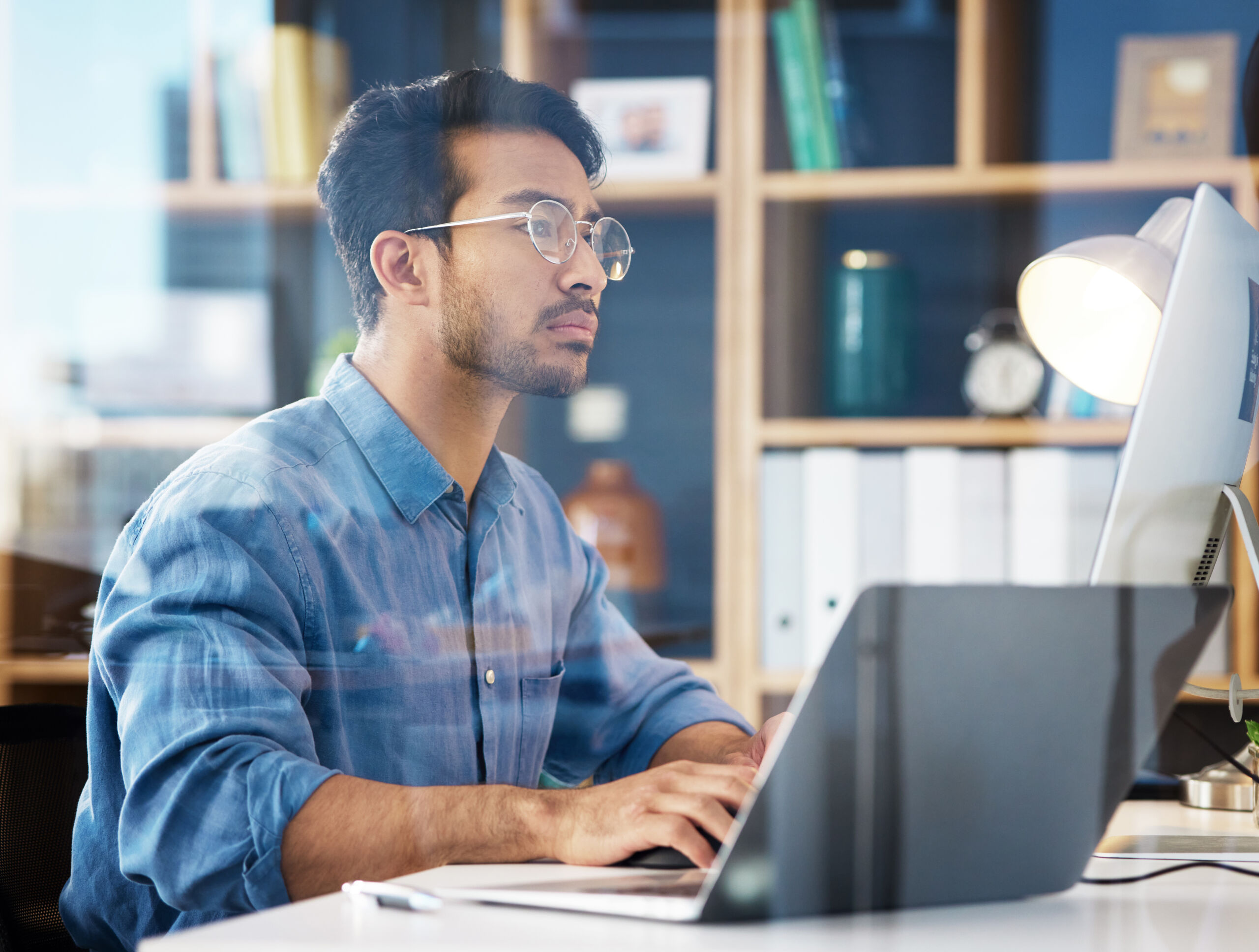
Trusted by federal agencies and leading primary contractors.





Machine Learning Services We Provide
1. Custom Machine Learning Model Development
2. Reinforcement Learning Services
3. Feature Engineering and Model Tuning
4. ML Integration
5. Computer Vision Services
6. Deep Learning Services
Case study
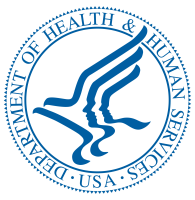
The U.S. Department of Health and Human Services (HHS) required advanced capabilities to predict trends and user behavior effectively across its various digital platforms. The existing analytical methods were insufficient for anticipating user needs and trends, which limited HHS’s ability to proactively adjust content and strategy to meet user expectations and improve engagement. Read the full case study.
Key Facts about Machine Learning Development
Enhanced Decision-Making: Machine learning (ML) provides tools to analyze vast amounts of data quickly and accurately, aiding in more informed decision-making for policy design and implementation. This ensures that policies are based on solid evidence and can address issues more effectively.
Predictive Capabilities: ML can predict outcomes and trends by uncovering patterns in historical data, enabling proactive measures in areas such as public health, crime prevention, and resource allocation. For example, predictive analytics can forecast disease outbreaks, allowing for timely intervention and resource distribution.
Efficiency Improvements: ML automates routine tasks and processes, leading to increased efficiency and allowing government employees to focus on higher-value work. This automation can reduce paperwork, streamline workflows, and improve overall productivity.
Improved Public Services: By leveraging ML, governments can enhance the quality and speed of public services, such as disaster response, healthcare, and social services, leading to better outcomes for citizens. For instance, ML can help in optimizing emergency response times and personalizing healthcare treatments.
Resource Optimization: ML helps in the equitable allocation of resources by identifying the areas and populations that need them the most, thereby optimizing public spending and maximizing impact. This ensures that limited resources are used where they are needed most, improving public welfare.
Complex Data Handling: Governments can utilize ML to process and analyze data from diverse sources, including social media and satellite imagery, to gain deeper insights and tackle complex issues more effectively. This capability is crucial for areas such as national security and environmental monitoring.
Support for Policymaking: ML models can simulate various policy scenarios, providing insights into potential impacts and helping policymakers craft more effective and equitable policies. These simulations can highlight unintended consequences and offer solutions before policies are implemented.
Definition: Machine Learning (ML) is a subset of artificial intelligence (AI) that focuses on the development of algorithms and statistical models that enable computers to perform tasks without explicit instructions, relying instead on patterns and inference. These systems learn from data, identify patterns, and make decisions with minimal human intervention.
How It Works: ML involves training a model using a large dataset, where the algorithm processes data inputs and adjusts its parameters to improve accuracy over time. This training can be supervised (with labeled data), unsupervised (with unlabeled data), or semi-supervised (a combination of both). Techniques like neural networks, decision trees, and clustering are commonly used in ML.
Applications: ML is used in various fields, such as natural language processing (NLP), computer vision, predictive analytics, and robotics. In the public sector, ML can enhance services like fraud detection, predictive maintenance, public safety, and personalized healthcare.
Benefits: The primary advantage of ML is its ability to handle and analyze large volumes of data quickly and accurately. It enables organizations to automate processes, predict trends, and make data-driven decisions, ultimately improving efficiency and effectiveness.
Challenges: Despite its benefits, ML poses challenges such as data privacy concerns, the need for large and high-quality datasets, potential biases in algorithms, and the requirement for specialized skills and infrastructure.
100s of federal offices, staff divisions, and contracting companies rely on our Data Science services.
Why Choose Analytics Logic for Machine Learning Services
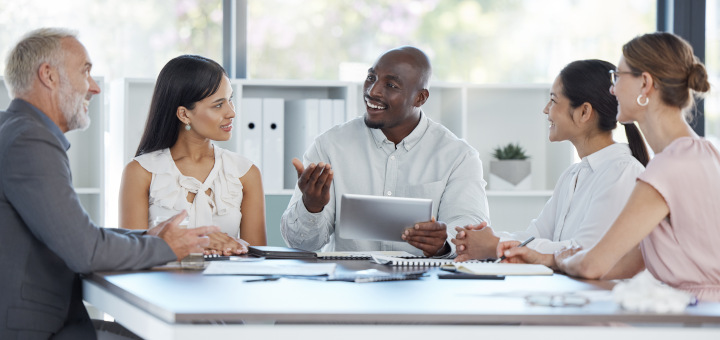
Deep Federal Experience
Benefit from our 12+ years of experience with U.S. Federal projects, ensuring compliance and deep understanding of governmental data needs and challenges.
Strategic Tech Partnerships
Leverage our vendor-neutral partnerships with top tech giants like Google, AWS, Azure, and more, allowing us to tailor cutting-edge data science solutions perfectly suited to your requirements.
Diverse Industry Insight
Our team of dedicated data experts brings invaluable cross-industry experience from government, healthcare, finance, and media, enabling us to cross-pollinate best practices across industries, providing you with innovative, tried-and-tested solutions that are not confined to a single perspective.
Our streamlined and effective process.
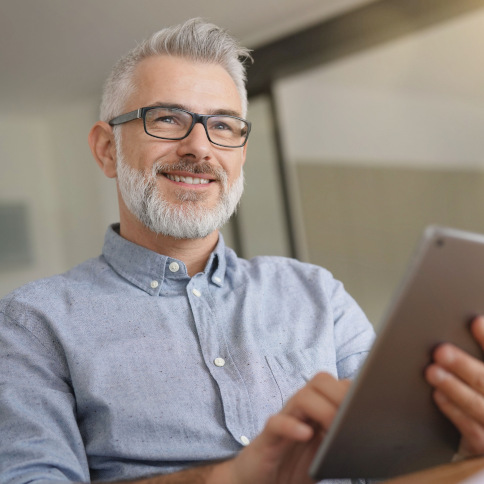
Step 1
Initiate discovery.
Step 2
Tailor solutions.
Step 3
Launch and execute.
Frequently Asked Questions (FAQ)
Artificial Intelligence (AI) is a broad field of computer science aimed at creating systems that can perform tasks requiring human intelligence, such as problem-solving, understanding natural language, recognizing patterns, and making decisions. Within AI, Machine Learning (ML) is a specific subset focused on developing algorithms and statistical models that allow computers to learn from and make predictions or decisions based on data. The key distinction lies in scope and functionality: AI encompasses a wide range of technologies and approaches, including ML, natural language processing (NLP), robotics, and expert systems, all aiming to perform various cognitive tasks.
ML, on the other hand, narrows its focus to data-driven algorithms that learn and improve over time. While AI systems can include rule-based or logic-based methods that do not necessarily involve learning from data, ML specifically requires a training process where systems are fed large amounts of data to learn patterns and enhance their predictions or decisions. This learning process is a defining feature of ML, enabling it to adapt and refine its performance based on data input.
Applications of AI and ML also differ. AI is used in a broad array of applications like autonomous vehicles, voice assistants, gaming, and robotics. In contrast, ML is particularly effective in areas such as predictive analytics, recommendation systems, image and speech recognition, and fraud detection. For example, an AI system like a voice assistant combines NLP, ML, and other AI techniques to understand and respond to user queries. Meanwhile, an ML application might be a recommendation system that suggests movies or shows based on a user’s viewing history and preferences.
Looking for reliable machine learning expertise for your agency?
See how we can help.
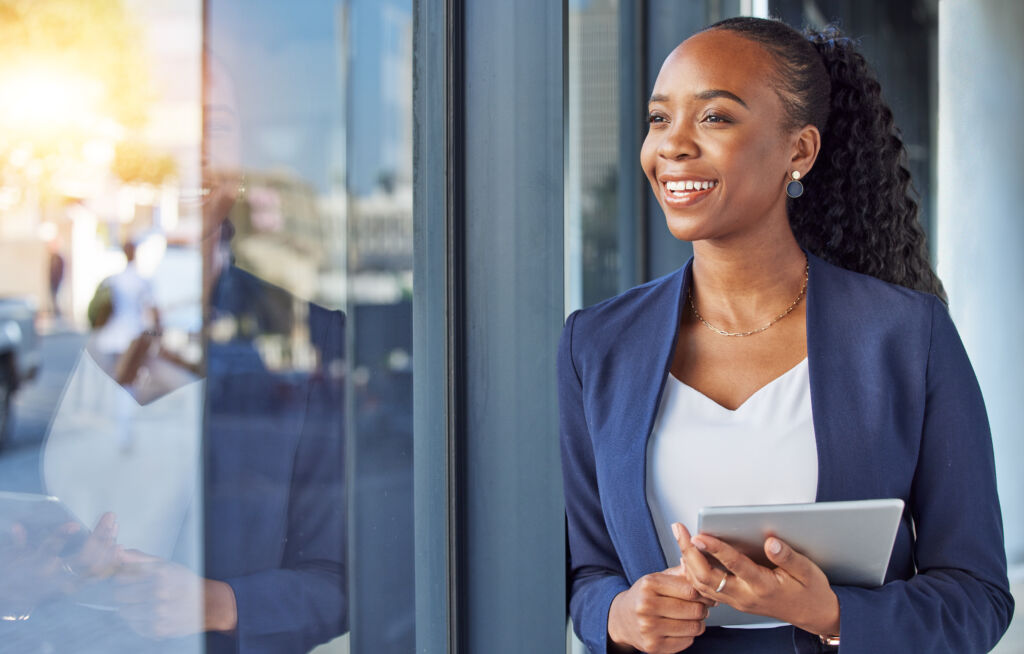